Translate this page into:
Utility of hematological parameters to predict tuberculosis disease among PLHIV: A retrospective cohort study
*Corresponding author: Parth Anilbhai Parmar, Department of Community Medicine, Shri M P Shah Government Medical College, Jamnagar, Gujarat, India. parthparmar6036@gmail.com
-
Received: ,
Accepted: ,
How to cite this article: Yogesh M, Vamja R, Makwana N, Parmar PA, Mody M, Lathiya MA, et al. Utility of hematological parameters to predict tuberculosis disease among PLHIV: A retrospective cohort study. J Lab Physicians. doi: 10.25259/JLP_244_2024
Abstract
Objectives
Tuberculosis (TB) remains a significant health challenge for people living with human immunodeficiency virus (PLHIV), highlighting the need for improved diagnostic and monitoring strategies. Hematological parameters, such as the monocyte-to-lymphocyte ratio (MLR), neutrophil-to-lymphocyte ratio (NLR), and anemia status, have emerged as potential biomarkers for TB disease in this vulnerable population. This present study aimed to assess the utility of hematological parameters in predicting TB disease among PLHIV attending an antiretroviral therapy (ART) center.
Materials and Methods
This retrospective cohort study was conducted in an ART center in a tertiary care hospital in Gujarat. Data from 813 PLHIV were analyzed, including hematological profiles, TB status, and demographic/clinical characteristics. TB was defined as either bacteriologically confirmed (through smear microscopy, GeneXpert Mycobacterium tuberculosis/rifampicin, or culture) or clinically diagnosed pulmonary TB based on suggestive symptoms and radiological findings.
Statistical analysis
Logistic regression models were developed to evaluate the association between hematological parameters and TB disease, adjusted for age, gender, baseline CD4 count, World Health Organization clinical stage, cotrimoxazole preventive therapy, isoniazid preventive therapy, and ART regimen.
Results
The proportion of TB-positive cases was substantially higher in the greater MLR group (49.4% vs. 10.3%). The logistic regression analysis revealed a strong association between a higher MLR and increased odds of having TB disease (adjusted odds ratio = 8.13, 95% confidence interval: 5.54–11.93, P < 0.001). Individuals with mild, moderate, or severe anemia had significantly higher odds of TB disease compared to those without anemia. The model incorporating MLR and anemia status demonstrated superior performance (area under the receiver operating characteristic curve [AUC] = 0.816, accuracy = 0.801) compared to the model with NLR and anemia status (AUC = 0.734, accuracy = 0.710), potentially reflecting the crucial role of monocytes in TB pathogenesis and granuloma formation in the context of human immunodeficiency virus coinfection.
Conclusions
This study highlights the potential utility of routinely available hematological parameters, particularly MLR and anemia status, as adjunctive tools for predicting TB disease in PLHIV. The integration of these cost-effective markers into existing diagnostic algorithms could enhance early detection, risk stratification, and targeted interventions.
Keywords
Anemia
Biomarkers
Hematological parameters
Human immunodeficiency virus
Monocyte-to-lymphocyte ratio
Tuberculosis
INTRODUCTION
Tuberculosis (TB) remains a formidable global health challenge, exacerbated by its deadly synergy with the human immunodeficiency virus (HIV) pandemic.[1] The compromised immune system in individuals living with HIV renders them highly susceptible to active TB disease, underscoring the urgent need for early diagnosis and prompt treatment.[2] However, conventional diagnostic methods for TB, such as clinical presentation, radiological findings, and microbiological tests, can be time-consuming, costly, and lack sensitivity, particularly in advanced immunosuppression.[3]
Hematological parameters, including hemoglobin levels, platelet counts, white blood cell differentials, and derived ratios, have emerged as potential markers for predicting TB and its monitoring.[4] Several studies have reported alterations in these parameters among people living with HIV (PLHIV) with active TB, such as anemia, thrombocytopenia, and leukocytosis.[5,6] These hematological abnormalities may reflect the underlying inflammatory response, immune dysregulation, and disease progression associated with TB and HIV co-infection.[7]
Among the hematological parameters, the monocyte-tolymphocyte ratio (MLR) and neutrophil-to-lymphocyte ratio (NLR) have garnered increasing attention as potential biomarkers for TB and other infectious diseases.[8,9] These ratios, derived from the differential white blood cell counts, may provide insights into the host’s immune response to TB infection and could aid in risk stratification and disease monitoring.[10]
In the context of HIV and TB co-infection, hematological parameters could serve as valuable adjuncts to existing diagnostic methods, particularly in resource-limited settings where access to advanced diagnostic tools is restricted.[11] Moreover, identifying potential hematological biomarkers associated with TB disease in PLHIV could contribute to our understanding of the pathogenesis and disease progression, ultimately informing strategies for early intervention and improved patient management.[12]
Several studies have explored the utility of hematological parameters in predicting TB disease and survival outcomes in PLHIV. For instance, Gatechompol et al. found that a higher MLR and lower hemoglobin levels were associated with an increased risk of TB after initiating antiretroviral therapy (ART).[13,14]
Despite these promising findings, there is a need for further research to validate the diagnostic and prognostic value of hematological parameters in different settings and populations. In addition, exploring the potential synergistic effects of combining multiple hematological markers could enhance the predictive accuracy for TB disease and survival outcomes in PLHIV.[15]
By incorporating the assessment of hematological parameters into existing HIV/TB programs, the National AIDS Control Program can leverage readily available and cost-effective tools to enhance early detection, risk stratification, and targeted interventions, ultimately contributing to improved management and outcomes for PLHIV with TB co-infection. With this context, the present study aimed to assess the utility of hematological parameters, such as hemoglobin levels, platelet counts, white blood cell differentials, and derived ratios (MLR and NLR), in predicting TB disease among PLHIV, and to evaluate the association between hematological parameters in PLHIV with TB co-infection, and also to investigate the potential of combining multiple hematological markers to enhance the predictive accuracy for TB disease in PLHIV.
MATERIALS AND METHODS
Study design
This retrospective cohort study was conducted at the ART center of a Tertiary Care Hospital in Gujarat. The study included data from ART patients registered from January 2018 to March 2024.
Study population
The study population consisted of individuals living with HIV who were attending the ART Centre.
Eligibility criteria
For inclusion criteria: HIV-positive individuals aged ≥18 years, enrolled in the ART program at the study center between January 2018 and March 2024, with complete baseline hematological data available including hemoglobin, white blood cell count, differential counts, and platelet count, and documented TB status (pulmonary TB [PTB] only; extrapulmonary TB cases were excluded) at baseline or during follow-up.
For exclusion criteria: Age below 18 years, incomplete or missing hematological data, unknown or indeterminate TB status, active malignancy, or other severe comorbidities that could significantly affect hematological parameters such as hematological disorders or chronic kidney disease, patients started on TB treatment before HIV diagnosis, and <6 months of follow-up data available.
TB definition and diagnosis
For this study, TB was defined as either bacteriologically confirmed or clinically diagnosed PTB.[16] Bacteriologically confirmed TB included cases with positive sputum smear microscopy for acid-fast bacilli (AFB), culture-positive for Mycobacterium TB complex, or positive GeneXpert Mycobacterium tuberculosis/rifampicin (MTB/RIF) assay. Clinically diagnosed TB cases were defined based on suggestive clinical features (such as persistent cough, fever, night sweats, and weight loss), radiological findings consistent with TB (cavities, infiltrates, pleural effusion on chest X-ray, or computed tomography scan), or strong clinical suspicion by a physician leading to initiation of a full course of anti-TB treatment. The diagnosis algorithm for TB in PLHIV included symptom screening at each visit using the World Health Organization (WHO) four-symptom screen (current cough, fever, night sweats, or weight loss). Symptomatic patients underwent sputum collection for AFB smear microscopy and GeneXpert MTB/RIF testing, along with chest X-ray. PLHIV with advanced HIV disease (CD4 count <200 cells/μL) or those not on ART underwent TB screening with GeneXpert MTB/RIF. Anti-TB treatment was initiated based on bacteriological confirmation or strong clinical suspicion, per national guidelines, with patients closely monitored for treatment response and adverse effects. In the present study, only PTB cases were included, with extrapulmonary TB excluded from the analysis.
The figure 1 shows the participant selection process [Figure 1].
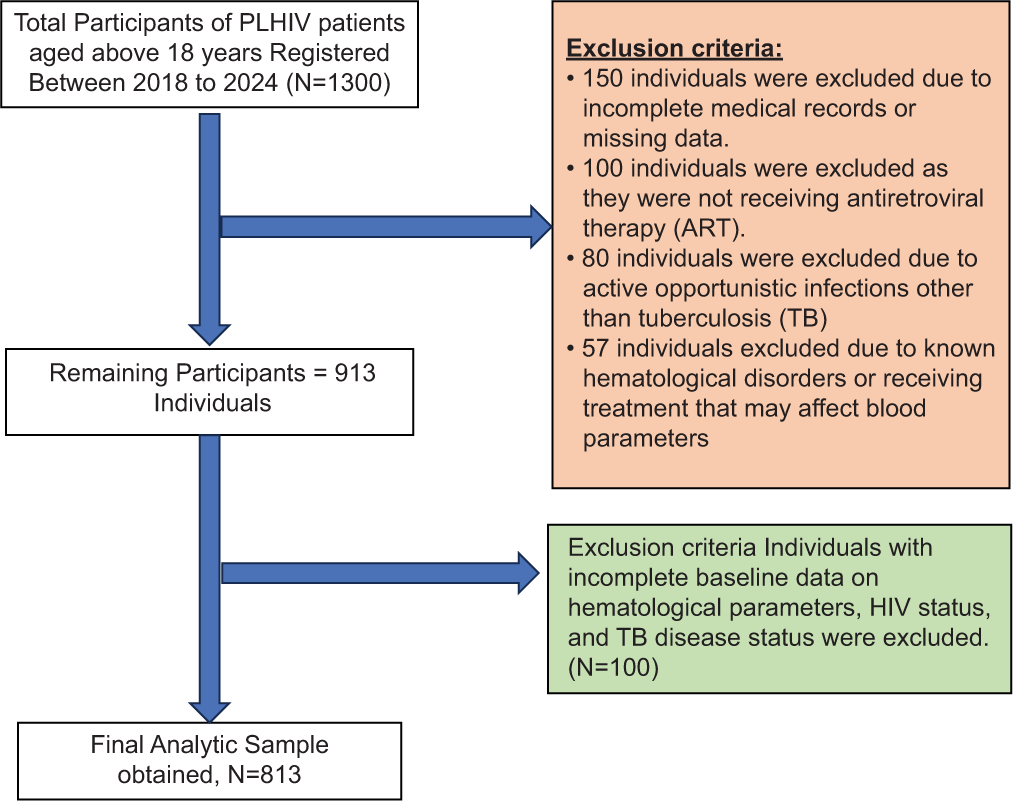
- Participants selection process. PLHIV: People living with human immunodeficiency virus, HIV: Human immunodeficiency virus
Data collection
The study utilized the participants’ existing medical records and laboratory data. Hematological parameters such as hemoglobin level, platelet count, white blood cell count, absolute neutrophil count, CD4 count, mean corpuscular volume (MCV), MLR, and NLR were extracted from the records. Information on the presence or absence of TB was also obtained.
MLR was stratified by the predefined cutoff of 0.23[13] for the overall population, MLR was stratified by the predefined cutoff of 0.23 for the overall population, with values >0.23 considered as greater MLR, and values ≤0.23 considered as lower MLR, based on previous studies, high NLR >2, and low NL ratio (NLR ≤2).[9]
Hemoglobin values at baseline visits were used. Categorization of anemia according to the WHO guideline criteria: [14]
No anemia: Hemoglobin ≥13 g/dL for men, ≥12 g/dL for women, mild anemia: hemoglobin 11–12.9 g/dL for men, 11–11.9 g/dL for women, moderate anemia: hemoglobin 8–10.9 g/dL for both men and women and severe anemia: hemoglobin <8 g/dL for both men and women.
Baseline characteristics such as age, gender, CD4 count, WHO clinical stage, preventive therapy received, ART regimen, and functional status (referring to the patient’s physical capability to perform daily activities, categorized as working, ambulatory, or bedridden) were collected for the study participants.
The functional status is categorized into three levels: Working: This category includes individuals who can engage in regular work or employment activities. They have a relatively high functional ability and can perform most daily activities independently. Ambulatory: This category refers to individuals who can walk or move around but may have some limitations in their mobility or ability to perform certain activities. They may require assistance or support for some tasks, but they are not completely bedridden. Bedridden: This category includes individuals who are confined to a bed or have severe limitations in their mobility and functional ability. They may require constant care and assistance for most activities of daily living.
Data quality assurance: Data extraction and validation: Established standardized protocols for data extraction from medical records, with clear guidelines for handling missing or incomplete data.
Data cleaning and quality checks: Perform regular data cleaning and quality checks to identify and address potential inconsistencies, outliers, or missing values in the dataset. This may involve range checks, logical consistency checks, and cross-validation with other data sources.
Data security and confidentiality: Implement robust data security measures to protect the confidentiality and integrity of the study data, including secure data storage, access controls, and deidentification procedures.
Statistical analysis
Descriptive statistics were used to summarize the baseline characteristics of the study population. Comparative analyses were performed to assess differences in hematological profiles between PLHIV with TB and those without TB. Student’s t-test or Mann–Whitney U-test was used for continuous variables, and Chi-square or Fisher’s exact test for categorical variables, depending on data distribution. The relationship between MLR and TB was analyzed by calculating baseline MLR values for all participants at their initial ART center visit, classifying cases as TB-positive based on either bacteriological confirmation or clinical diagnosis during the study period, documenting the temporal sequence (specifically recording whether TB diagnosis occurred after the baseline MLR measurement), and calculating the crude incidence rate of TB for patients with high versus low MLR. Logistic regression models were developed to assess the predictive ability of hematological parameters, particularly MLR and anemia status, for the presence of TB. Odds ratios (ORs) with corresponding 95% confidence intervals (CIs) were calculated to quantify the strength of associations, with P < 0.05 considered statistically significant. Model performance was evaluated using accuracy, specificity, sensitivity, and area under the receiver operating characteristic curve (AUC). All statistical analyses were performed using Jamovi statistical software, with adjustments made for potential confounders, including age, gender, baseline CD4 count, WHO clinical stage, preventive therapies, and ART regimen.
RESULTS
Table 1 provides the baseline characteristics of the study population, which consisted of 813 individuals. The mean age of the participants was 39.7 ± 13.7 years. The majority of the participants were male (67.7%). Most of the participants (79%) had a CD4 count >350 cells/μL, and 87.6% were in WHO Clinical Stage 1. Around 41.5% of the participants received cotrimoxazole preventive therapy (CPT), and 70% received isoniazid preventive therapy (IPT). The majority (97%) were on a first-line ART regimen. The mean baseline hemoglobin level was 11.5 ± 2.48 g/dL. Regarding anemia status, 32.3% had no anemia, 26.9% had mild anemia, 31.9% had moderate anemia, and 8.9% had severe anemia. Most of the participants (92.4%) had a working functional status [Table 1].
Characteristic | Frequencies | Percentages |
---|---|---|
Age (years), mean±SD | 39.7±13.7 | |
Gender, n (%) | ||
Male | 550 | 67.7 |
Female | 263 | 32.3 |
CD4 count (cells/μL) | ||
≤350 | 171 | 21 |
>350 | 642 | 79 |
WHO clinical stage, n (%) (n=806) | ||
Stage 1 | 706 | 87.6 |
Stage 2 | 1 | 0.1 |
Stage 3 | 46 | 5.7 |
Stage 4 | 53 | 6.6 |
CPT given, n (%) | 338 | 41.5 |
IPT given, n (%) | 571 | 70 |
ART regimen, n (%) | ||
First line | 789 | 97 |
Second line | 21 | 2.5 |
Other | 3 | 0.5 |
Baseline hemoglobi n (g/dL), mean±SD | 11.5±2.48 | |
Anemia status, n (%) | ||
No anemia | 263 | 32.3 |
Mild anemia | 219 | 26.9 |
Moderate anemia | 259 | 31.9 |
Severe anemia | 72 | 8.9 |
Functional status, n (%) (n=807) | ||
Working | 746 | 92.4 |
Ambulatory | 34 | 4.2 |
Bedridden | 27 | 3.3 |
SD: Standard deviation, IQR: Interquartile range, CPT: Cotrimoxazole preventive therapy, IPT: Isoniazid preventive therapy, ART: Antiretroviral therapy, WHO: World health organization, CD4: Cluster of Differentiation 4
Table 2 shows a comparison of hematological profiles of PLHIV patients with and without TB. This table compares the hematological profiles of PLHIV with PTB and without PTB. The mean hemoglobin level was found to be lower in PLHIV with PTB (10.2 ± 2.1 g/dL) compared to those without PTB (11.9 ± 2.43 g/dL). The mean platelet count and mean CD4 count were almost marginally similar between the two groups (P = 1.00 and 0.643). PLHIV with TB had a higher mean white blood cell count (7.06 ± 3.62 × 103/μL vs. 6.5 ± 2.4 × 103/μL, P = 0.011) and a higher mean absolute neutrophil count (4.61 ± 2.9 × 103/μL vs. 3.78 ± 1.94 × 103/μL, P value < 0.001) compared to those without TB. PLHIV with TB had a lower mean MCV (79 ± 9.9 vs. 82.3 ± 11.07, P = 0.001) and higher median MLR ratio (0.286 ± 0.274 vs. 0.158 ± 0.151, P = 0.001) and NLR ratio (2.60 ± 2.26 vs. 1.71 ± 1.16, P = 0.001) compared to those without TB [Table 2]. Of the 813 total participants, 310 had greater MLR (>0.23) while 503 had lower MLR (≤0.23). Among those with greater MLR, 153 (49.4%) were TB positive, and 157 (50.6%) were TB negative. In contrast, among those with lower MLR, only 52 (10.3%) were TB positive, while 451 (89.7%) were TB negative. This difference in TB positivity between MLR groups was statistically significant (χ2 = 155, df = 1, P < 0.001).
Parameter | PLHIV with PTB (n=205) | PLHIV without PTB (n=608) | P-value |
---|---|---|---|
Hemoglobi n (g/dL), mean±SD | 10.2±2.1 | 11.9±2.43 | 0.643 |
Platelet count (×109/L), mean±SD | 273.9±135 | 296±132 | 1.00 |
White blood cell count (×103/μL), mean±SD | 7.06±3.62 | 6.5±2.4 | 0.011* |
Absolute Neutrophil count (×103/μL), mean±SD | 4.61±2.9 | 3.78±1.94 | <0.001** |
CD4 count (cells/μL), mean±SD | 414±287 | 425.9±323 | 0.643 |
MCV, mean±SD | 79±9.9 | 82.3±11.07 | 0.001** |
MLR ratio, median±IQR | 0.286±0.274 | 0.158±0.151 | 0.001** |
NLR ratio, median±IQR | 2.60±2.26 | 1.71±1.16 | 0.001** |
SD: Standard deviation, IQR: Interquartile range, PLHIV: People living with human immunodeficiency virus; PTB: Pulmonary tuberculosis, SD: Standard deviation, IQR: Interquartile range, g/dL: grams per deciliter, L: Liter, μL: microliter, MCV: Mean corpuscular volume, MLR: Monocyte to lymphocyte ratio, NLR: Neutrophil to lymphocyte ratio, CD4: Cluster of differentiation 4.*P<0.05 statistically significant **P<0.001 highly statistically significant
Table 3 provides modeling analysis using logistic regression analysis and prediction analysis. This table presents the results of two logistic regression models for predicting the presence of TB [Table 3].
Predictor | COR (95% CI) | aOR (95% CI) | P-value |
---|---|---|---|
MLR status | |||
Greater vs. Less | 11.93 (8.28–17.18) | 8.13 (5.54–11.93) | <0.001 |
Anemia status | |||
Mild versus no anemia | 3.44 (1.95–6.07) | 3.03 (1.69–5.44) | <0.001 |
Moderate versus no anemia | 8.58 (5.02–14.65) | 7.19 (4.13–12.52) | <0.001 |
Severe versus no anemia | 9.22 (4.56–18.65) | 7.75 (3.77–15.94) | <0.001 |
Model fit: McFadden’s R2=0.242, Adjusted R2=0.229 Model performance: AUC=0.816, Accuracy=0.801, Sensitivity=0.698, Specificity=0.836. COR: Crude odds ratio, aOR: Adjusted odds ratio, CI: Confidence interval, MLR: Monocyte-to-lymphocyte ratio, PLHIV: People living with human immunodeficiency virus, AUC: Area under the ROC curve. Adjusted for age, gender, baseline CD4 count, WHO clinical stage, cotrimoxazole preventive therapy, isoniazid preventive therapy, and antiretroviral therapy regimen
Model 1
In this model, the predictors were MLR status (greater vs. less) and anemia status (mild, moderate, and severe vs. no anemia). The model had an R2 of 0.242, indicating that it explained 24.2% of the variation in the outcome. The odds of having TB were 8.13 times higher for individuals with a greater MLR ratio compared to those with a lower MLR ratio (95% CI: 5.54–11.93, P < 0.001). Compared to those without anemia, the odds of having TB were 3.03 times higher for those with mild anemia (95% CI: 1.69–5.44, P < 0.001), 7.19 times higher for those with moderate anemia (95% CI: 4.13–12.52, P < 0.001), and 7.75 times higher for those with severe anemia (95% CI: 3.77–15.94, P < 0.001). The model had an accuracy of 80%, an AUC of 81.6% with a specificity of 83.6%, and a sensitivity of 69.8%.
Model 2
Model 2 aimed to predict the TB status among PLHIV using hematological parameters, especially anemia status (mild, moderate, and severe vs. no anemia) and NLR ratio (high vs. low). The model had an R2 of 0.122, indicating that it explained 12.2% of the variation in the outcome. Compared to those without anemia, the odds of having TB were 3.72 times higher for those with mild anemia (95% CI: 2.13– 6.49, P < 0.001), 7.35 times higher for those with moderate anemia (95% CI: 4.35–12.42, P < 0.001), and 7 times higher for those with severe anemia (95% CI: 3.59–13.63, P < 0.001). Individuals with a high NLR ratio had 2.27 times higher odds of having TB than those with a low NLR ratio (95% CI: 1.62–3.20, P < 0.001). The model had an accuracy of 71%, an AUC of 73.4%, a specificity of 75.8%, and a sensitivity of 56.6% [Table 4].
Predictor | COR (95% CI) | aOR (95% CI) | P-value |
---|---|---|---|
NLR status | |||
High versus low | 2.80 (2.04–3.86) | 2.27 (1.62–3.20) | <0.001 |
Anemia status | |||
Mild versus no anemia | 3.94 (2.29–6.77) | 3.72 (2.13–6.49) | <0.001 |
Moderate versus no anemia | 8.19 (4.93–13.60) | 7.35 (4.35–12.42) | <0.001 |
Severe versus no anemia | 8.12 (4.25–15.51) | 7.00 (3.59–13.63) | <0.001 |
Model fit: McFadden’s R2=0.122, Adjusted R2=0.110 Model performance: AUC=0.734, Accuracy=0.710, Sensitivity=0.566, Specificity=0.758. COR: Crude odds ratio, aOR: Adjusted odds ratio, CI: Confidence interval, NLR: Neutrophil-to-lymphocyte ratio, AUC: Area under the ROC curve. Adjusted for age, gender, baseline CD4 count, WHO clinical stage, cotrimoxazole preventive therapy, isoniazid preventive therapy, and antiretroviral therapy regimen
Figure 2 shows the receiver operating characteristic curves of Model 1 (MLR and Anemia) [Figure 2] and Figure 3 presents the curves for Model 2 (NLR and Anemia)[Figure 3], both used for predicting tuberculosis in PLHIV.

- Receiver operating characteristic curves of model-1 (monocyte-to-lymphocyte ratio and anemia) for predicting tuberculosis in people living with human immunodeficiency virus.
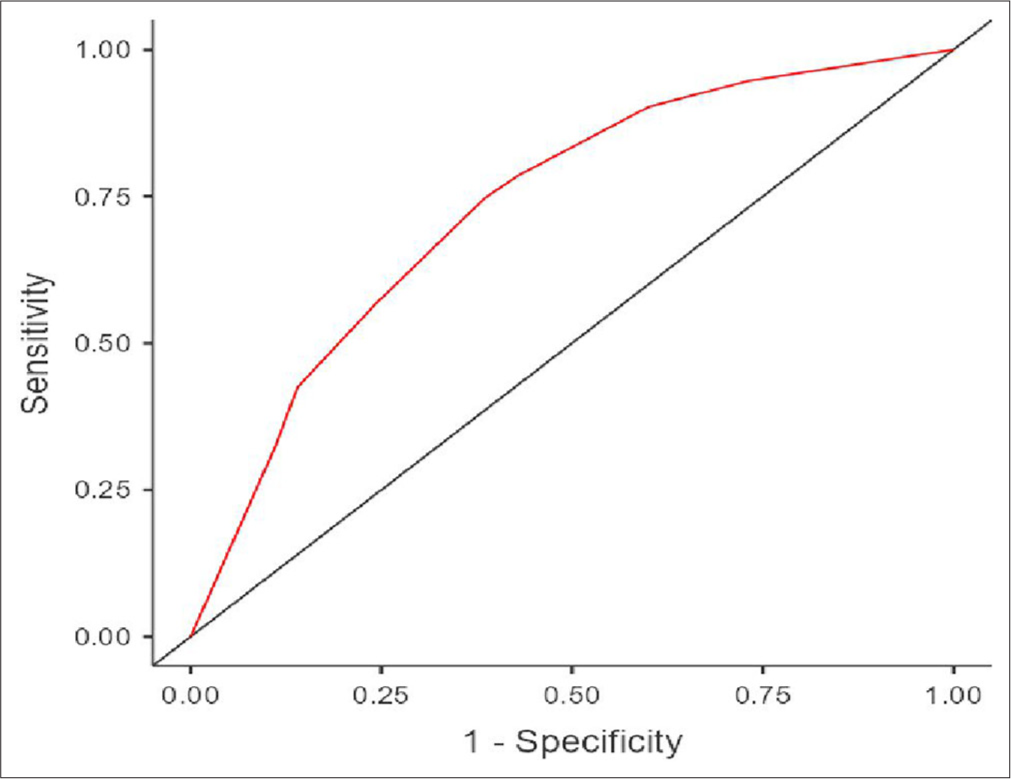
- Receiver operating characteristic curves of model-2 (neutrophil-to-lymphocyte ratio and anemia) for predicting tuberculosis in people living with human immunodeficiency virus.
DISCUSSION
This retrospective cohort study aimed to assess the utility of hematological parameters in predicting TB disease among PLHIV attending an ART center. The findings of this study contribute to a growing body of evidence highlighting the potential value of routinely available hematological markers in the management of HIV and TB co-infection.
A key finding of this study was the significant association between the MLR and the presence of TB disease in PLHIV. The logistic regression analysis (Model 1) revealed that individuals with a higher MLR ratio had an 8-fold increased odds of having TB compared to those with a lower MLR ratio (adjusted OR = 8.13, 95% CI: 5.54–11.93, P < 0.001). This is consistent with previous studies that have reported an elevated MLR in PLHIV with active TB disease.[7,8,17] The MLR is a derivative of the differential white blood cell count, reflecting the balance between monocytes and lymphocytes. An elevated MLR may indicate an ongoing inflammatory response and immune dysregulation associated with TB infection.[18,19]
The study also examined the NLR as a potential biomarker for predicting TB disease in PLHIV. In Model 2, individuals with a high NLR (>2) had 2.27 times higher odds of having TB compared to those with a low NLR (95% CI: 1.62–3.20, P < 0.001). This finding is consistent with previous studies that have reported an elevated NLR in PLHIV with active TB disease.[9,16] The NLR reflects the balance between neutrophils and lymphocytes, with an elevated ratio indicating an ongoing inflammatory response and immune dysregulation.[18]
While both MLR and NLR are derived from the differential white blood cell count, the observed difference in their predictive performance for TB disease in PLHIV is noteworthy. Model 1, which incorporated MLR and anemia status, demonstrated superior model fit (McFadden’s R2 = 0.242) and predictive performance (AUC = 0.816, accuracy = 0.801) compared to Model 2, which included NLR and anemia status (McFadden’s R2 = 0.122, AUC = 0.734, accuracy = 0.710). This suggests that the MLR may be a more robust predictor of TB disease in PLHIV than the NLR.
Another key finding of this study was the strong association between anemia and TB disease in PLHIV. The logistic regression models revealed that individuals with mild, moderate, or severe anemia had significantly higher odds of having TB compared to those without anemia (Model 1 and Model 2). These results align with previous research indicating a high prevalence of anemia among PLHIV with active TB disease.[5,14,20,21]
The observed association between anemia and TB disease in PLHIV can be explained by several potential mechanisms. Chronic inflammation and immune activation associated with HIV and TB co-infection can lead to anemia through various pathways, including impaired erythropoiesis, increased red blood cell destruction, and blood loss.[6] In addition, nutrient deficiencies, which are common in PLHIV, can contribute to the development of anemia.[11] Anemia may also be an indicator of disease severity and advanced immunosuppression, which could increase the risk of TB reactivation or progression.[12]
The observed differences in other hematological parameters, such as white blood cell counts, absolute neutrophil counts, and red blood cell parameters (e.g., MCV) between PLHIV with and without TB, further support the potential diagnostic utility of these readily available markers. In the present study, PLHIV with TB had significantly higher mean white blood cell counts (7.06 ± 3.62 × 10^3/μL vs. 6.5 ± 2.4 × 10^3/μL, p-value = 0.011) and mean absolute neutrophil counts (4.61 ± 2.9 × 10^3/μl vs. 3.78 ± 1.94 × 10^3/μl, P < 0.001) compared to those without TB.[4,7,18] In addition, PLHIV with TB had a lower mean MCV (79 ± 9.9 vs. 82.3 ± 11.07, P value = 0.001), which may indicate a higher prevalence of microcytic anemia in this group.[22,23]
These findings collectively highlight the potential value of routinely available hematological parameters, particularly the MLR and anemia status, as adjunctive tools in the diagnosis and management of TB disease in PLHIV. While further research is needed to validate and refine these findings, the integration of these cost-effective markers into existing diagnostic algorithms and clinical decision-making processes could potentially improve case detection, risk stratification, and targeted interventions for this vulnerable patient population, especially in resource-limited settings.
Limitations
Retrospective study design: The retrospective nature of the study relies on existing medical records and data, which may be subject to potential biases and inaccuracies in documentation. Single-center study: While these findings from a single tertiary care center in Gujarat may have limited generalizability across different geographical and demographic contexts, they provide important preliminary evidence for the utility of hematological parameters in TB prediction among PLHIV. Potential confounding factors: While the study adjusted for some potential confounders, there may be other unmeasured or unaccounted factors that could have influenced the observed associations. Lack of longitudinal data: The study may have benefited from longitudinal data on hematological parameters and clinical outcomes to better understand the dynamic changes and their prognostic value over time.
Despite these limitations, the strengths of this study include the relatively large sample size of 813 PLHIV and the comprehensive analysis of multiple hematological parameters of TB disease. The utilization of routinely available laboratory data also highlights the potential practical applicability of these findings in resource-limited settings, where access to more advanced diagnostic tools may be limited. There is a lack of data on important confounding factors, including serum albumin, globulin, A/G ratio, diabetes status, and other opportunistic infections, all of which could significantly impact hematological parameters and TB risk. Future studies should incorporate these variables for more comprehensive analysis.
In summary, this study provides compelling evidence for the potential utility of hematological parameters, particularly the MLR and anemia status, in predicting TB disease among PLHIV. The strong associations observed between these hematological markers and TB disease underscore their potential as adjunctive tools in the management of HIV and TB co-infection.
Future prospective studies, incorporating additional biomarkers and clinical outcomes, are warranted to further validate and refine the use of hematological parameters in the diagnosis, monitoring, and risk stratification of PLHIV with TB disease. Moreover, the integration of these readily available and cost-effective markers into existing diagnostic algorithms and clinical decision-making processes could potentially improve the overall management and outcomes for this vulnerable patient population, especially in resource-limited settings.
CONCLUSIONS
This retrospective cohort study has provided valuable insights into the potential utility of routinely available hematological parameters in predicting TB among PLHIV. The findings highlight the strong associations between MLR, anemia, and the presence of TB disease in this vulnerable population.
However, several limitations should be acknowledged. The retrospective design limits causal inference and may introduce information bias. As a single-center, hospital-based study, our findings may not be generalizable to the broader PLHIV population across different settings. In addition, we were unable to account for all potential confounders, such as detailed nutritional status, certain co-infections, and ART adherence that might influence both hematological parameters and TB risk.
Despite these limitations, by incorporating the assessment of hematological parameters into existing HIV/TB programs, healthcare providers can leverage readily available and cost-effective tools to enhance early detection, risk stratification, and targeted interventions. Future prospective, multi-center studies with comprehensive assessment of confounding factors are warranted to validate these findings before widespread implementation. Nevertheless, this study provides a foundation for the potential role of hematological markers in improving the management and outcomes for this vulnerable patient population.
Author’s contribution
YM, RV, PP: Conceived and designed the study; RV, PP: Collected and analyzed the data; YM, NM: Assisted in data interpretation and statistical analysis; MM, ML, AT: Contributed to drafting and revising the manuscript. All authors reviewed and approved the final version of the manuscript.
Ethical approval
The research/study was approved by the Institutional Review Board at M.P. Shah Govt. Medical College and Guru Gobind Singh Hospital, number 284/03/2023, dated 22nd February 2024.
Declaration of patient consent
Patient’s consent not required as patients identity is not disclosed or compromised.
Conflicts of interest
There are no conflicts of interest.
Use of artificial intelligence (AI)-assisted technology for manuscript preparation
The authors confirm that there was no use of artificial intelligence (AI)-assisted technology for assisting in the writing or editing of the manuscript and no images were manipulated using AI.
Financial support and sponsorship: Nil.
References
- Tuberculosis and HIV co-infection. PLoS Pathog. 2012;8:e1002464.
- [CrossRef] [PubMed] [Google Scholar]
- Malaria and tuberculosis co-infection-A review. Oxf Open Immunol. 2023;4:iqad008.
- [CrossRef] [PubMed] [Google Scholar]
- Hematological abnormalities of pulmonary tuberculosis patients with and without HIV at the university of Gondar hospital, Northwest Ethiopia: A comparative cross-sectional study. Tuberc Res Treat. 2018;2018:5740951.
- [CrossRef] [PubMed] [Google Scholar]
- Prevalence of anemia among people living with HIV: A systematic review and meta-analysis. EClinicalMedicine. 2022;44:101283.
- [CrossRef] [PubMed] [Google Scholar]
- Anemia, iron status, and HIV: A systematic review of the evidence. Adv Nutr. 2020;11:1334-63.
- [CrossRef] [PubMed] [Google Scholar]
- Ratio of monocytes to lymphocytes in peripheral blood identifies adults at risk of incident tuberculosis among HIV-infected adults initiating antiretroviral therapy. J Infect Dis. 2014;209:500-9.
- [CrossRef] [PubMed] [Google Scholar]
- Predicting tuberculosis at antiretroviral therapy initiation: The combination of monocyte-to-lymphocyte ratio and hemoglobin level may be a key. AIDS. 2023;38:115-7.
- [CrossRef] [PubMed] [Google Scholar]
- Predicting the risk of pulmonary tuberculosis based on the neutrophil-tolymphocyte ratio at TB screening in HIV-infected individuals. BMC Infect Dis. 2019;19:667.
- [CrossRef] [PubMed] [Google Scholar]
- The association between the ratio of monocytes: Lymphocytes and risk of tuberculosis among HIV infected postpartum women. J Acquir Immune Defic Syndr. 2014;67:573-5.
- [CrossRef] [PubMed] [Google Scholar]
- Anemia in HIV infection: Clinical impact and evidence-based management strategies. Clin Infect Dis. 2004;38:1454-63.
- [CrossRef] [PubMed] [Google Scholar]
- The prevalence of anemia among human immunodeficiency virus-infected individuals in East Africa: A systematic review and meta-analysis. Medicine (Baltimore). 2023;102:e33810.
- [CrossRef] [PubMed] [Google Scholar]
- Monocyte to lymphocyte ratio and hemoglobin level to predict tuberculosis after antiretroviral therapy initiation. AIDS. 2023;38:31-8.
- [CrossRef] [PubMed] [Google Scholar]
- Prevalence and risk factors of anaemia in hospitalised HIV-infected patients in southeast china: A retrospective study. Epidemiol Infect. 2019;147:e81.
- [CrossRef] [PubMed] [Google Scholar]
- Evaluation of prognostic significance of hematological profiles after the intensive phase treatment in pulmonary tuberculosis patients from Romania. PLoS One. 2021;16:e0249301.
- [CrossRef] [PubMed] [Google Scholar]
- Higher neutrophil-lymphocyte ratio in TB/HIV co-infection compared to pulmonary tuberculosis. Indones Biomed J. 2021;13:375-82.
- [CrossRef] [Google Scholar]
- Monocyte-to-lymphocyte ratio as a predictor of TB among people living with HIV. Int J Tuberc Lung Dis. 2021;25:933-8.
- [CrossRef] [PubMed] [Google Scholar]
- Neutrophil-to-monocyte-plus-lymphocyte ratio as a potential marker for discriminating pulmonary tuberculosis from nontuberculosis infectious lung diseases. Lab Med. 2019;50:286-91.
- [CrossRef] [PubMed] [Google Scholar]
- Monocyte-to-lymphocyte ratio is associated with tuberculosis disease and declines with anti-TB treatment in HIV-infected children. J Acquir Immune Defic Syndr. 2019;80:174-81.
- [CrossRef] [PubMed] [Google Scholar]
- Anemia and anti-tuberculosis treatment outcome in persons with pulmonary tuberculosis: A multi-center prospective cohort study. J Infect Public Health. 2023;16:974-80.
- [CrossRef] [PubMed] [Google Scholar]
- Relationship between anemia and systemic inflammation in people living with HIV and tuberculosis: A sub-analysis of the CADIRIS clinical trial. Front Immunol. 2022;13:916216.
- [CrossRef] [PubMed] [Google Scholar]
- Hematological and biochemical parameters in apparently healthy Indian population: Defining reference intervals. Indian J Clin Biochem. 2014;29:290-7.
- [CrossRef] [PubMed] [Google Scholar]
- Causes of moderate and severe anaemia in a high-HIV and TB-prevalent adult population in the Eastern cape province, South Africa. Int J Environ Res Public Health. 2023;20:3584.
- [CrossRef] [PubMed] [Google Scholar]